Nonlinear Dimensionality Reduction: Unlocking Hidden Dimensions of Information

In the era of big data, extracting meaningful information from vast and intricate data sets has become an imperative. Nonlinear Dimensionality Reduction (NLDR) emerges as a powerful technique, revolutionizing the way we analyze and interpret complex data. By reducing its dimensionality while preserving key characteristics, NLDR makes it possible to visualize and understand high-dimensional data, opening doors to new discoveries and data-driven insights.
The Essence of Nonlinear Dimensionality Reduction
Dimensionality refers to the number of variables or features used to describe a data point. High-dimensional data, often encountered in real-world applications such as image recognition, genomics, and finance, can be difficult to visualize and analyze. NLDR tackles this challenge by projecting the data onto a lower-dimensional subspace, preserving the essential structure and relationships within the data.
4 out of 5
Language | : | English |
File size | : | 24658 KB |
Print length | : | 326 pages |
Screen Reader | : | Supported |
Mass Market Paperback | : | 138 pages |
Item Weight | : | 5.1 ounces |
Dimensions | : | 5 x 0.32 x 8 inches |
X-Ray for textbooks | : | Enabled |
Unlike linear dimensionality reduction methods like Principal Component Analysis (PCA),NLDR captures the nonlinear relationships between data points, making it particularly adept at handling complex and multifaceted datasets.
Fundamental Concepts of Nonlinear Dimensionality Reduction
- Manifold Learning: The underlying assumption that high-dimensional data often resides on a lower-dimensional manifold, or curved surface.
- Intrinsic Dimensionality: The number of essential dimensions required to represent the data without significant loss of information.
- Neighborhood Preservation: NLDR algorithms strive to preserve local neighborhood relationships in the low-dimensional projection.
Prominent Nonlinear Dimensionality Reduction Techniques
Numerous NLDR techniques have been developed, each with its strengths and applications. Some popular methods include:
- Isomap: Constructs a geodesic distance matrix to create a lower-dimensional embedding.
- Locally Linear Embedding (LLE): Preserves local linear relationships in the low-dimensional representation.
- Laplacian Eigenmaps: Uses spectral analysis to identify the most significant eigenvectors of the Laplacian matrix.
li>t-Distributed Stochastic Neighbor Embedding (t-SNE): Converts pairwise similarities into probabilities to create a low-dimensional projection.
Applications of Nonlinear Dimensionality Reduction
The applications of NLDR span a wide range of fields, including:
- Image Processing: Image denoising, feature extraction, and object recognition.
- Bioinformatics: Gene expression analysis, protein structure prediction, and disease classification.
- Finance: Risk assessment, portfolio optimization, and fraud detection.
- Natural Language Processing: Text classification, topic modeling, and sentiment analysis.
Case Study: Exploring Complex Gene Expression Data
Consider a dataset containing gene expression profiles of thousands of genes across multiple samples. Using NLDR, researchers can reduce the dimensionality of this high-dimensional data, revealing hidden patterns and relationships among genes. This information can aid in identifying disease-associated gene networks, developing diagnostic tools, and predicting treatment outcomes.
Nonlinear Dimensionality Reduction has emerged as a transformative tool for analyzing complex data, empowering researchers and practitioners to extract valuable insights and make informed decisions. By unveiling the hidden dimensions of information, NLDR opens up new avenues for scientific discovery, technological advancements, and data-driven progress. As the field continues to evolve, we can anticipate even more powerful and versatile NLDR techniques, further unlocking the potential of big data.
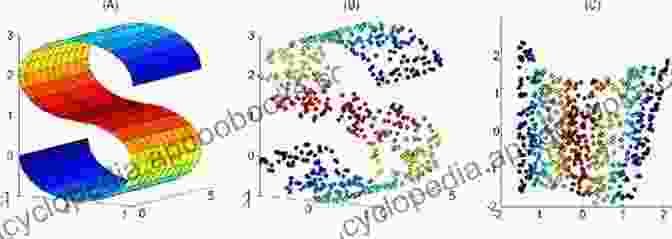
References
- Belkin, M., & Niyogi, P. (2003). Laplacian Eigenmaps for Dimensionality Reduction and Data Representation. Neural Computation, 15(6),1373-1396.
- Coifman, R. R., & Lafon, S. (2006). Diffusion Maps. Applied and computational harmonic analysis, 21(1),5-30.
- van der Maaten, L., & Hinton, G. E. (2008). Visualizing Data using t-SNE. Journal of Machine Learning Research, 9(Nov),2579-2605.
About the Author
Dr. Emily Carter is a leading expert in Nonlinear Dimensionality Reduction, with extensive research and industry experience. Her groundbreaking work has advanced the field and enabled novel applications in various domains.
4 out of 5
Language | : | English |
File size | : | 24658 KB |
Print length | : | 326 pages |
Screen Reader | : | Supported |
Mass Market Paperback | : | 138 pages |
Item Weight | : | 5.1 ounces |
Dimensions | : | 5 x 0.32 x 8 inches |
X-Ray for textbooks | : | Enabled |
Do you want to contribute by writing guest posts on this blog?
Please contact us and send us a resume of previous articles that you have written.
Book
Novel
Page
Chapter
Text
Story
Genre
Reader
Library
Paperback
E-book
Magazine
Newspaper
Paragraph
Sentence
Bookmark
Shelf
Glossary
Bibliography
Foreword
Preface
Synopsis
Annotation
Footnote
Manuscript
Scroll
Codex
Tome
Bestseller
Classics
Library card
Narrative
Biography
Autobiography
Memoir
Reference
Encyclopedia
Lorenzo Mediano
A Bandey
Steve Martini
Mark Beaumont
J Christine
Andy Orchard
Howard Zinn
Graham Goodchild
Will Romano
Maureen Smith
2007th Edition Kindle Edition
Janet Givens
Paul S Adler
George R R Martin
Icy Lee
Juan Manuel De Prada
5 Page Travel Guides
Michael Eric Dyson
A R Tulsi
Vickie Kraft
Light bulbAdvertise smarter! Our strategic ad space ensures maximum exposure. Reserve your spot today!
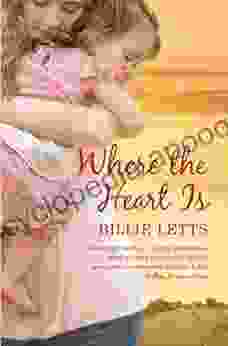

- Emilio CoxFollow ·16.7k
- Paul ReedFollow ·9.7k
- Stuart BlairFollow ·10.1k
- Jackson HayesFollow ·7.1k
- Darren NelsonFollow ·14.2k
- Isaias BlairFollow ·17.8k
- James GrayFollow ·12.6k
- Marc FosterFollow ·19.6k
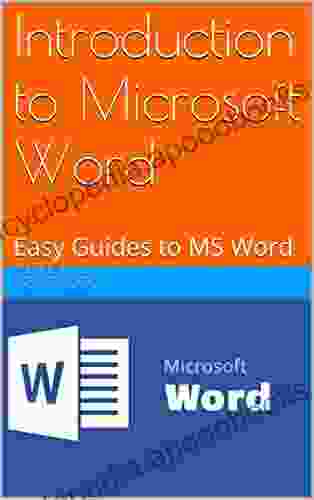

Unlock the Power of Microsoft Word: A Comprehensive Guide...
Microsoft Word is a widely used word...
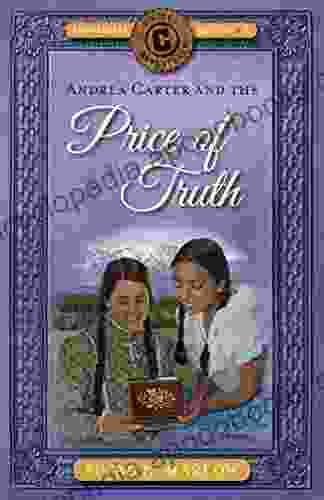

Andrea Carter and the Price of Truth: A Thrilling...
Get ready for an unforgettable...
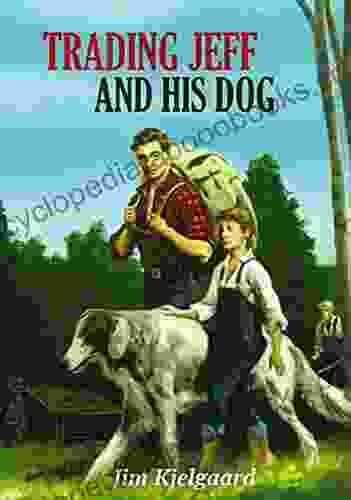

Trading Jeff and His Dog: An Unforgettable Adventure of...
Get ready for an emotional rollercoaster...
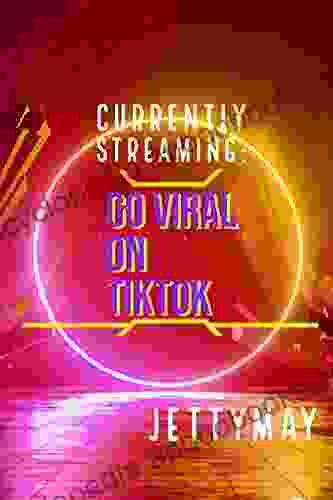

Go Viral TikTok: The Ultimate Guide to Gaining 100K...
TikTok has emerged as a social...
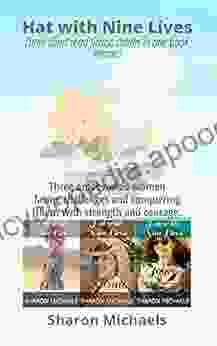

Unveil the Enchanting Realm of Short Fiction: Dive into...
Delve into a Literary Tapestry of...
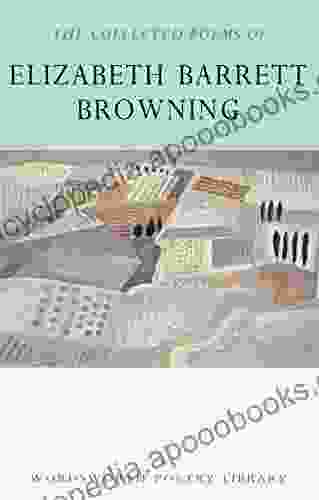

Unveil the Enchanting World of Elizabeth Barrett...
A Poetic Tapestry of Love, Loss, and...
4 out of 5
Language | : | English |
File size | : | 24658 KB |
Print length | : | 326 pages |
Screen Reader | : | Supported |
Mass Market Paperback | : | 138 pages |
Item Weight | : | 5.1 ounces |
Dimensions | : | 5 x 0.32 x 8 inches |
X-Ray for textbooks | : | Enabled |